Computational Analysis and Acceleration Research Group
Biomedical and UAV related research
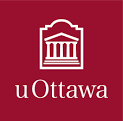
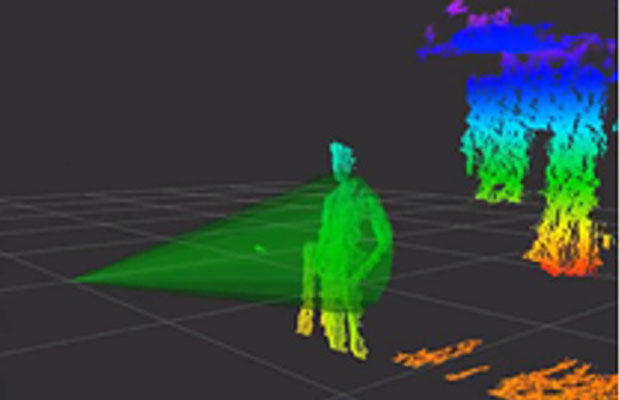
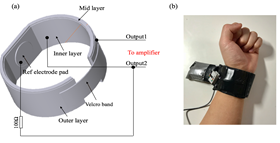
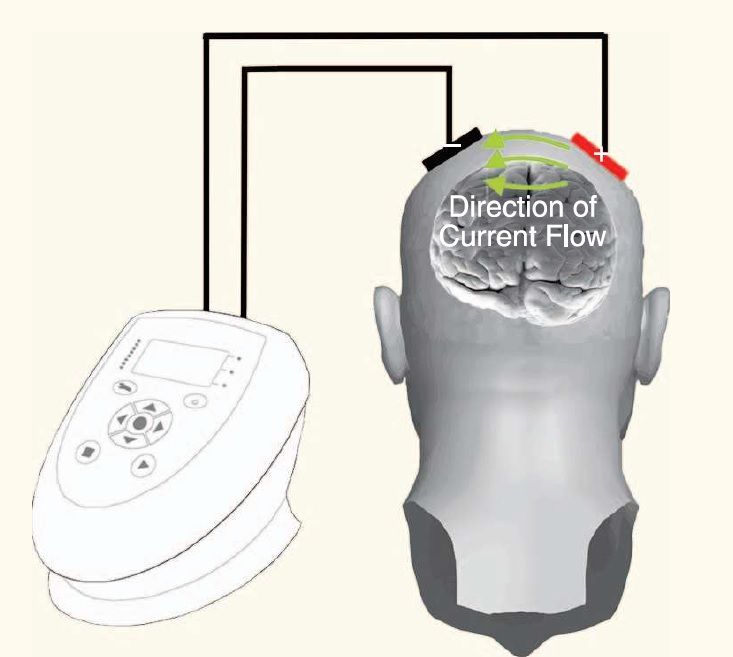
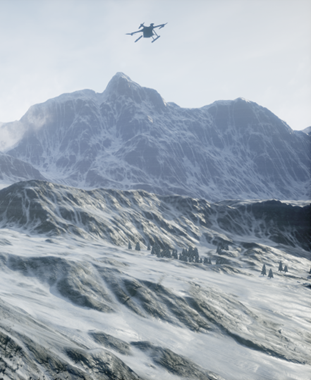
Biomedical and UAV related research
About
At Computational Analysis and Acceleration Research Group (CARG), we develop data-driven solutions to tackle real-world challenges in biomedical, communications and surveillance applications. We combine physical models with machine learning models that account for uncertainty, ensuring our systems are robust and ready for real-life conditions. Our researchers are involved in all stages of the processing pipeline, from experiment design and data collection to data processing and the development of machine learning models for our applications, including UAV detection and inspection, as well as patient data for wearable and contactless medical solutions. To date, more than 70 graduate students and postdoctoral researchers have been part of our multidisciplinary team.
Areas of Focus
History: this research group evolved from Computer Architecture research group (original CARG). Computer architecture research group was formed in 2008 and focused on the following topics: computer architectures, signal processing architectures, hardware and software accelerators, GPU architectures and programming and algorithmic modifications for more efficient implementation in hardware. In recent years our research has gone in several different directions and therefore we decided to change the name of our group to Computational Analysis and Acceleration Research Group (new CARG).
Computational analysis combines aspects of mathematical modeling, data processing, and algorithm development to produce insights that guide research and practical applications.
Acceleration refers to techniques and technologies used to increase the speed of data processing and computation.
New project: Modeling and Simulations for Intruder UAS Detection based for Interceptor UAS agents, supported by NRC has just started. The project will be completed by 2028.
The newly published study introduces an innovative imaging system that monitors cardiopulmonary patients without physical contact, capturing subtle neck movements that correlate with vital physiological parameters. This advancement could significantly improve continuous monitoring capabilities while enhancing patient comfort and reducing healthcare-associated infection transmission in clinical environments.
The study presents new mathematical approaches to improve the accuracy and reliability of continuous cuffless blood pressure estimation technologies. These novel metrics enable healthcare providers to better track blood pressure fluctuations over time without the discomfort and limitations of conventional cuff-based measurements. This advancement could significantly enhance long-term cardiovascular monitoring, allowing for more personalized treatment strategies and earlier intervention in hypertension-related conditions.